©Aleksandr Rumiantsev – stock.adobe.com
Surgery or no surgery?
Personalised care for hearing impaired people
In Germany, about one in five people suffers from impaired hearing, and among the over-70s it is as high as one in two. Worldwide, the WHO estimates the number of people affected to be 1.5 billion, and by 2050 it is expected to be almost 2.5 billion. Impaired hearing can severely affect the lives of those affected, for example in their jobs or in their participation in society, and thus also has an impact on their psychological well-being. Electronic hearing prostheses, which are surgically implanted in the cochlea, are a promising treatment option for severe hearing loss. These cochlear implants (CIs) are intended to improve the quality of life for patients for whom a conventional hearing aid is no longer sufficient. However, a CI does not help all patients equally well. This discourages many hearing-impaired people – even those who would benefit from the operation. It would therefore be helpful for those affected and for the doctors treating them to be able to assess the likelihood of success of the operation as accurately as possible in advance. The interdisciplinary project “Understanding Cochlear Implant Outcome Variability using Big Data and Machine Learning Approaches” aims to make exactly this possible. Scientists at L3S are working together with colleagues from the German Hearing Centre (DHZ), the Technical University of Braunschweig and the Hannover Medical School (MHH) to personalise the care of hearing-impaired patients with cochlear implants and thus enable more successful surgeries.
In fact, there are large individual differences in hearing after a CI implantation. “The causes are manifold and not well understood in detail so far. Therefore, our approach aims to use artificial intelligence (AI) to predict the outcome of a potential surgery in order to select the best individual therapy for each patient,” says project collaborator Johanna Schrader, who is writing her doctoral thesis on this topic at L3S.
Previous prediction models consider comparatively small data sets with few patients and are limited to clinical data. “This is probably why it has not yet been possible to fully uncover which factors influence CI therapy success,” says Schrader. And presumably that is why no AI prediction model is used in clinical practice yet.
As the world’s largest competence centre for cochlear implantations, the DHZ in Hanover has an extensive data set of over 10,000 CI implantations. In the project, the researchers analyse a wide variety of data types: in addition to clinical and genetic data, image data from CT scans and measurements of metabolic products. At L3S, Johanna Schrader and her colleague Dr Sowmya Sundaram are using this data to develop a machine learning model that can predict the individual chances of success of an operation for each individual patient. At the same time, the model provides information about which factors were particularly relevant for the prognosis. “During development, we constantly verify the results in close cooperation with researchers at the DHZ and integrate requirements such as transparency and interpretability directly into the model. With colleagues from the MHH, we are expanding the data basis of the AI model to include genetic information from the patients,” explains Schrader.
At the same time, the scientists are establishing common data standards within the project. For example, the L3S is working together with the DHZ to structure the causes of hearing loss hierarchically. Such standards will facilitate future data analyses and add additional expertise to the databases. The predictions of the developed model can be used directly on site at the DHZ and help medical professionals to plan a tailor-made therapy for each individual hearing-impaired patient.
Contact
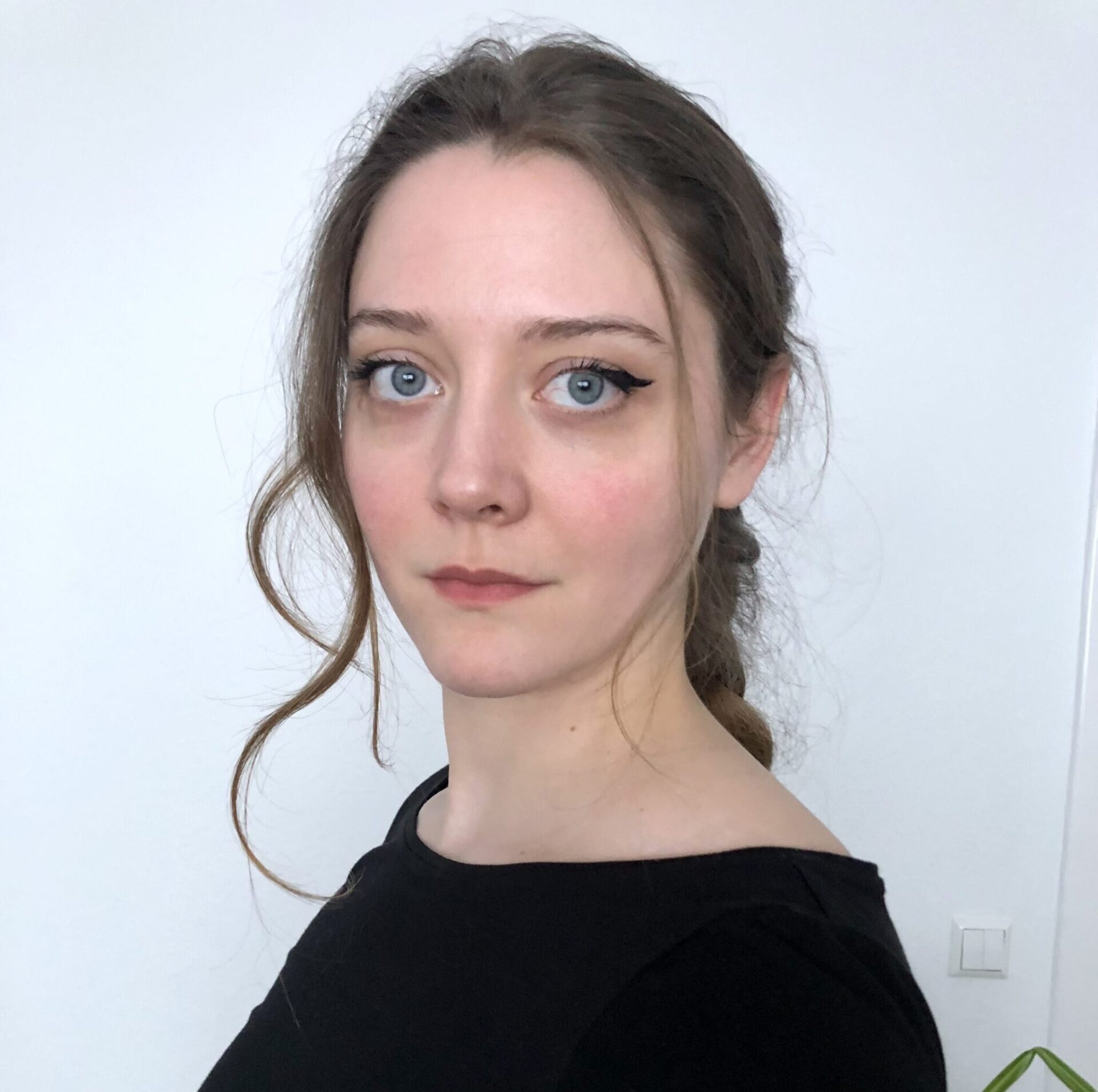
Johanna Schrader
Johanna Schrader is a PhD student at L3S and works on predictive models and data analysis in the CI project. Her research focuses on machine learning and causal methods for personalised medicine.