Foto: Adobe Stock
On the way to individual therapy
Every human being is genetically unique. Nevertheless, the treatment of diseases is still not very individual. Even individual genetic variants can reduce the effectiveness of a therapy. Individual treatment therefore requires genetic testing and analysis of specific genetic variations that may affect a patient’s suitability for a particular drug. However, not only genes, but also characteristics such as family history, lifestyle habits and concomitant diseases influence the results of a therapy and must be taken into account in decision-making.
The necessary knowledge for a targeted individual therapy, as aimed at by precision medicine, is encoded in large amounts of biomedical data (big data). AI-based analysis tools evaluate the data and uncover patterns that correlate with treatment effectiveness and disease progression. In the EU-funded projects iASiS and BigMedilytics, Prof. Dr. Maria-Esther Vidal and her team from L3S are working together with clinicians, geneticists, computer scientists, lawyers and decision-makers from other European institutions to gain knowledge for precision medicine from data and to improve health care in Europe through Big Data.
Knowledge from heterogeneous data
The scientists are faced with a number of challenges: Biomedical data are presented in countless formats that have to be combined for the optimal therapy decision, e.g. medical records, image or genome data. The different structure and quality of the data are the main obstacles to knowledge acquisition. In addition, the results of machine learning algorithms must be explainable and transparent, especially when decisions about individualized therapies are based on predictions and discovered patterns. In both projects, the Vidal team is developing new data-driven infrastructures, in particular algorithmic solutions for the problems of big data integration, large-scale query processing and decision support.
Individual treatment of lung cancer and Alzheimer’s disease
iASiS uses Big Data to advance precision medicine for two life-threatening diseases: lung cancer and Alzheimer’s disease. The iASiS data-driven infrastructure extracts knowledge from scientific literature, electronic health records, medical images, genomic data and pharmacogenomic databases and uses biomedical ontologies to describe the knowledge gained and integrate it into the iASiS knowledge graphs. New machine learning algorithms based on the iASiS knowledge graph identify patterns that correlate with long-term survival and disease progression. The iASiS infrastructure not only supports physicians in the individual diagnosis and treatment of patients, but also simplifies cooperation with political decision-makers. The aim is for policy makers to be able to define appropriate measures to identify and reduce disease risks at an early stage.
Better health care
BigMedilytics also integrates Big Data and combines data-driven strategies for personalized diagnosis and treatment. In addition, BigMedilytics aims to strengthen the sustainability of healthcare systems across Europe by reducing costs while improving quality and access to care. The BigMedilytics Big Data Healthcare Analytics Blueprint describes ways in which Big Data solutions can be used in the healthcare sector throughout Europe in compliance with data protection and ethics regulations.
iASiS and BigMedilytics expand the repertoire of calculation methods that support conscientious diagnosis and treatment. In the near future, diseases that cause the deaths of millions of people will be able to be treated individually. In this way, the chances of survival and the quality of life of those affected can be increased to a maximum.
Contact
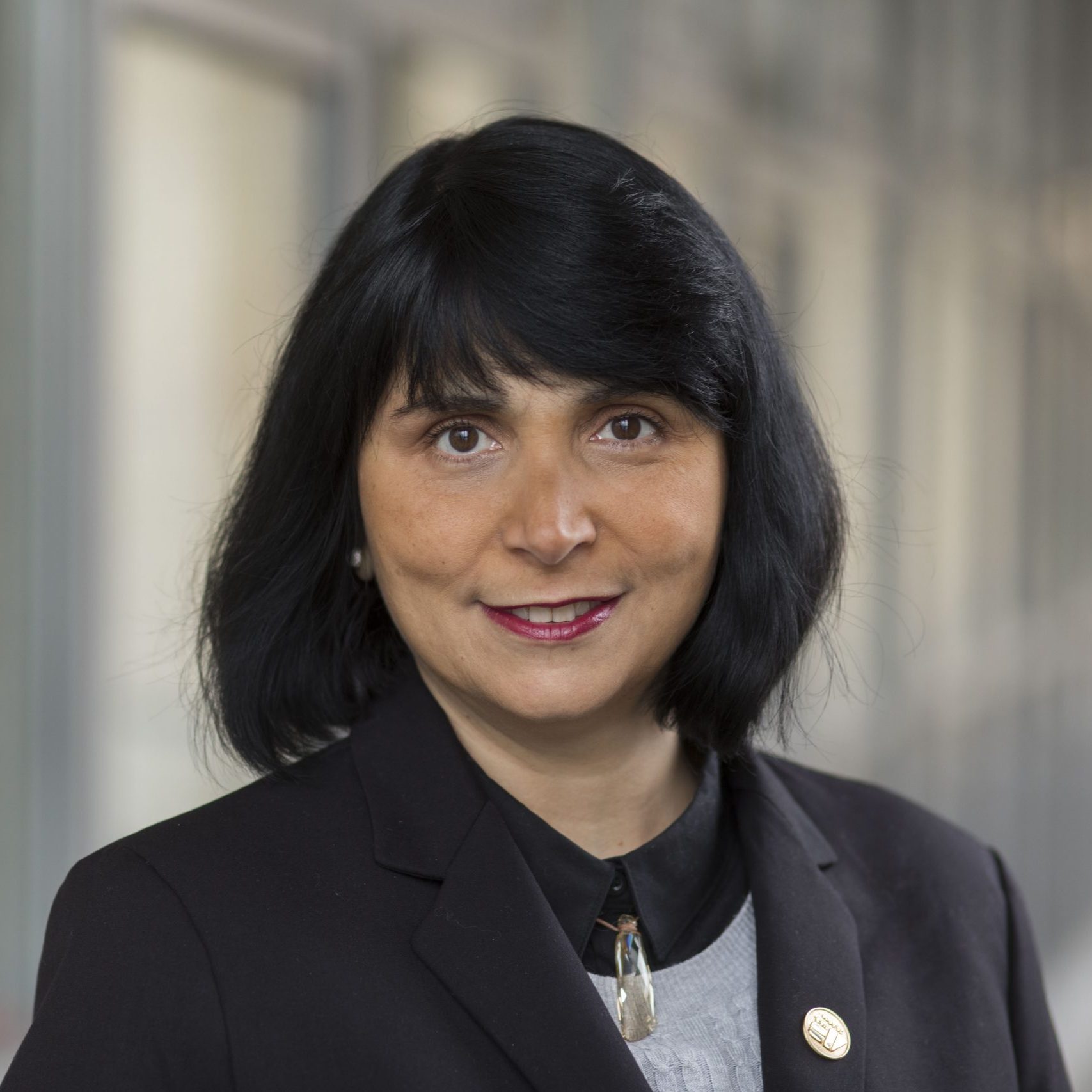
Prof. (Univ. Simon Bolivar) Dr. Maria-Esther Vidal
Maria-Esther Vidal is head of the Scientific Data Management group at the Leibniz Information Centre for Technology and Natural Sciences (TIB) and a member of the L3S. She leads the development of calculation methods for big data integration, large-scale query processing and knowledge discovery.