Foto: ©Adobe Stock 79707649 – stock.adobe.com
AI identifies factors for therapeutic success
New chances against breast cancer
Every year, about 70,000 women in Germany develop breast cancer. Almost every eighth woman is affected at some point, and a third of them are younger than 55. Researchers are still trying to develop successful therapies to cure this disease. One of these methods is called neoadjuvant therapy. This treatment is given to the patient before surgery in order to reduce the size of the tumour and improve the initial situation for surgery or to be able to operate in a breast-conserving way. After completion of the therapy, the shrunken tumour is removed. The treatment was successful if no tumour cells can be detected in the tissue samples from the breast and the lymph nodes. This is called pathological complete remission (pCR).
However, breast cancer is a heterogeneous disease that manifests itself differently in patients. Accordingly, the treatment results are also inconsistent, and the therapy does not show the desired success in every case. In the breast cancer network of the Leibniz AI Laboratory, scientists from the L3S and the Hannover Medical School (MHH) want to find out which factors are decisive for the success of the therapy.
The researchers first jointly examined patient data and highlighted characteristics that could help predict a patient’s therapy outcome. After cleaning and processing the data set, data from 260 patients are available so far, each with 16 relevant variables, including mutated genes, tumour size and number of affected lymph nodes.
The L3S scientists Can Aykul and Wadhah Zai El Amri are working on the project with artificial intelligence methods. They apply classification methods such as decision trees and other complex algorithms to predict the value of pathological complete remission. “With the current data set, we achieve an accuracy of about 75 per cent,” says Zai El Amri. “Also, the data is modelled as a graph to put it into a knowledge graph.” This, he says, connects the data and makes it easier to identify statistically related phenomena. By combining the graph model with the classification methods, the accuracy of the prediction can be further increased.
Currently, the researchers are in the process of uncovering the causal relationships between the variables. They also want to use causal research to understand why some patients benefit from neoadjuvant therapy and others do not. In the end, the AI system should help to find the right cancer therapy for each patient.
Contact
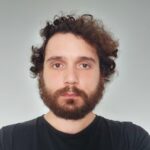
Can Aykul
Can Aykul is a doctoral student and research assistant at the Leibniz AI Lab.
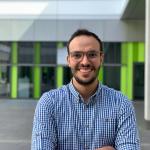
Wadhah Zai El Amri
Wadhah Zai El Amri is a doctoral student and research assistant at the Leibniz AI Lab.