The tool Kl as a protective shield against the misuse of Kl. Stable Diffusion XL for the prompt: “A protective shield made of binary code fends off threatening fake news, expressive painting in blue and turquoiser.
Social Media and Society
Disinformation: Opportunities and Dangers of Artificial Intelligence
In the era of the internet and social media, the spread of false and misleading information (fake news) and derogatory and hateful posts (hate speech) has become a problem for democracy. Disinformation and deep fakes can often only be identified with great effort, even by experts and fact-checking organisations such as Snopes or PolitiFact. Possible consequences of such posts: Manipulation of opinions, a loss of trust in news channels, the division of society. Artificial intelligence (AI) can help experts to check posts for misinformation and disinformation. However, AI also has the potential for misuse in this context.
Diverse dissemination methods
A significant challenge in detecting fake news is the wide variety of methods that can be used to create misleading or harmful content. These range from disseminating false “facts” in texts to manipulating or creating (deep fakes) images, graphics, or videos to posts that threaten, insult, or defame individuals or groups. False or disinformation can also be spread via the way news is communicated. In videos, events can be distorted simply by changing the order or deliberately withholding individual scenes. The second challenge is that facts are often complicated to verify, for example, if only subtle changes are made, or the facts are not yet clear, as is the case with very recent events. False information can often only be refuted once it has gone viral.
Misuse of AI
Artificial intelligence methods are an essential tool in the fight against fake news. But generative AI models can also have the opposite effect: For example, malicious actors could misuse them to produce convincing fake news content, i.e. real-looking news articles, tweets or videos. For instance, Generative Adversarial Networks (GANs) are used to generate deep fakes. The training process of these GANs is characterised by the fact that such content is not only generated but also classified, for example, as real or false. This simultaneous and mutual optimisation improves the generator and the detector during training.
In some cases, deceptively real deep fakes are created, which, in conjunction with speech synthesis methods, can generate videos with false statements from public figures. Some examples have been publicised in the media: In 2022, Berlin’s then-mayor, Franziska Giffey, was the victim of a video call. The caller was allegedly Kiev’s mayor, Vitali Klitschko.
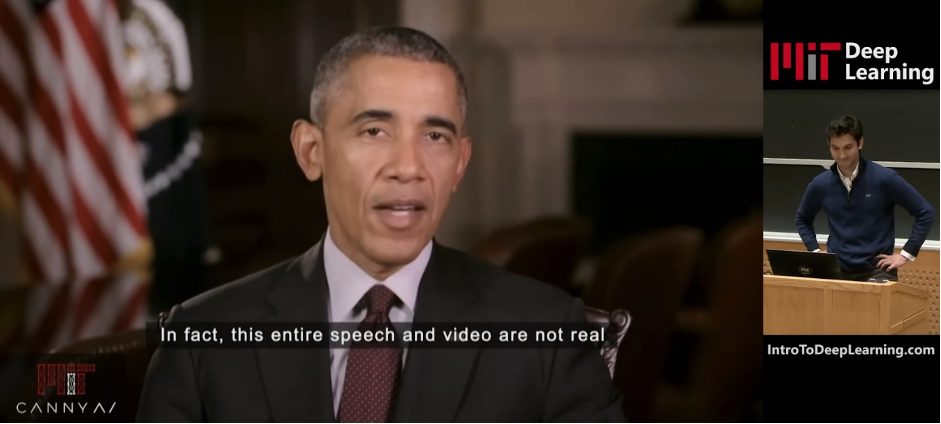
Deep Fake of Barack Obama as an introduction to the deep-learning lecture at MIT (Massachusetts Institute of Technology). ©Alexander Amini and Ava Amini, “MIT Introduction to Deep Learning”, source: http://introtodeeplearning.com/
Watch the complete video
Generative AI methods have become widely known since the publication of Chat-GPT. Generative AI models can generate natural texts for various tasks, such as summaries or translations. One risk is that the generated text may not be entirely truthful. This behaviour can be deliberately exploited, but it can also unintentionally lead to the spread of supposedly credible fake news.
Media, AI, and fake news
In the fight against fake news, AI approaches that analyse text, image, audio, and video information unimodally and multimodally are needed. Most of them come from the field of deep learning. Natural language processing methods, in conjunction with knowledge graphs or other sources of information, are used to verify claims and identify posts with hateful content or polarising viewpoints. In the Social Media and Climate Change project, L3S scientists use such methods to categorise social media posts regarding their stance on climate change and identify disinformation.
Computer vision approaches can recognise image manipulation and – in combination with audio analysis, among other things – even deep fakes. Multimodal AI methods can also be used to analyse messages consisting of images and text as well as video and audio, for example, concerning specific narrative patterns or matching information in pictures and text. In the FakeNarratives project, machine learning methods are combined with techniques from the digital humanities and discourse analysis to investigate disinformation narratives in news videos.
In addition to developing innovative AI methods, it is necessary to sensitise the population to all forms of fake news and the use of AI. The European data innovation hub MediaFutures, coordinated at L3S, has supported start-ups, companies and artists in developing digital products, services and artworks to counteract disinformation and strengthen the media literacy of the population.
Work in the digital society
It is not only social media and digital platforms that strongly influence social development. Digitalisation is also changing the world of work enormously. The Future Lab Society & Work looks at the consequences, effects and opportunities for shaping the future. The focus here is on the responsible use of digital technologies. The L3S is primarily concerned with the robustness and fairness of AI models. Another important research topic is participation in IT projects as a critical factor for successful IT projects in the long term and, therefore, for sustainable digitalisation.
Contact
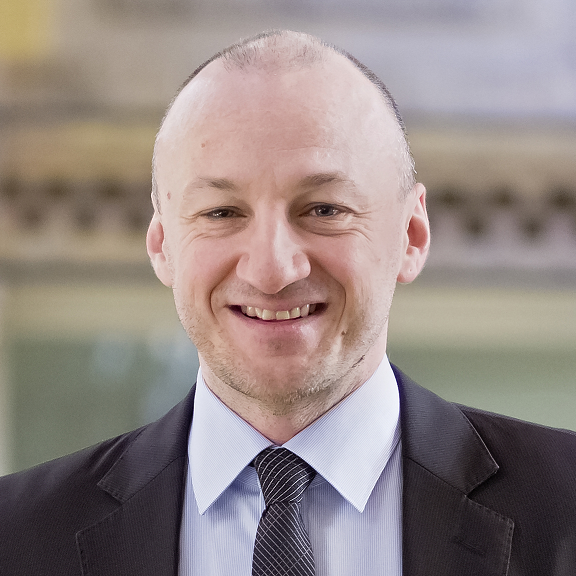
Prof. Dr. Ralph Ewerth
Ralph Ewerth is head of the Visual Analytics research group of the Joint Lab of L3S and TIB (Leibniz Information Center for Science and Technology) and a Professor of Visual Analytics at LUH.
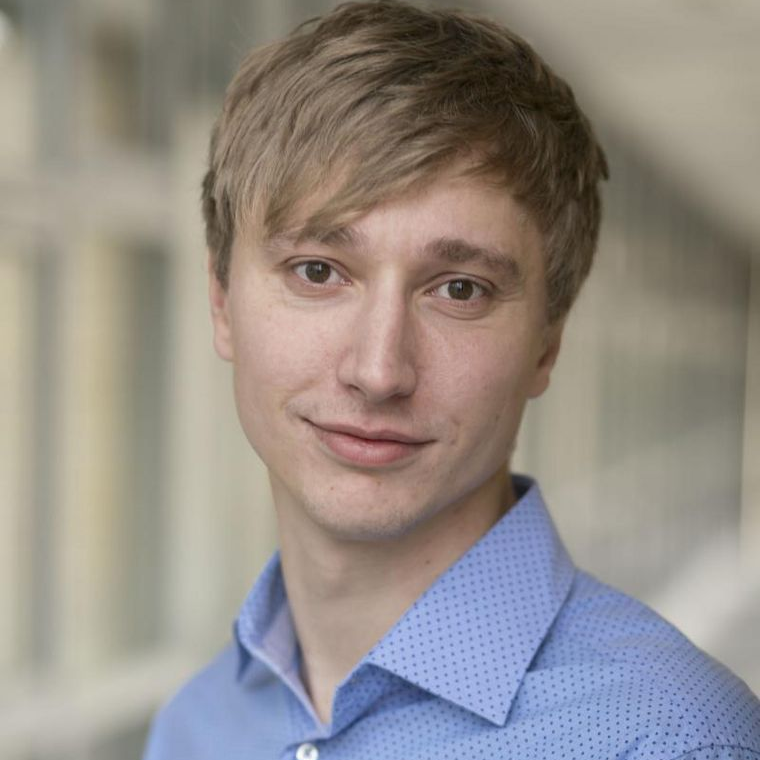
Dr.-Ing. Eric Müller-Budack
Eric Müller-Budack is a postdoctoral researcher in the Visual Analytics research group of the Joint Lab of L3S and TIB (Leibniz Information Center for Science and Technology). His research focuses on computer vision and multimedia information retrieval.