©Egor – stock.adobe.com
Legal implications of machine learning
Data protection, transparency and responsibility
When we talk about machine learning (ML), one fact is evident, even almost trivial: to train algorithms, data is needed. Algorithms can successfully recognize patterns on the basis of large amounts of data and thus expand their “knowledge”. It is questionable to whom these data “belong”, whether exclusive rights exist in them and whether they are suitable as an asset. However, neither the legal construction of the property nor the intellectual property rights apply to data. Possible solutions are currently part of the scientific discourse.
Who owns the data?
Data from autonomous vehicles is an example that illustrates the different interests involved. Software companies, car manufacturers and other service providers would like to have exclusive access to this data because it promises new business models. The affected drivers of autonomous vehicles, on the other hand, are concerned about the protection of their privacy. In particular, it will be better protected by the basic Regulation on data protection, which will come into force on 25 May. Here, information duties and rights of those affected are just as firmly anchored as principles of transparency, purpose limitation and data minimisation. In the ABIDA project, L3S lawyers, together with partners from other disciplines, are trying to develop solutions that balance the different interests.
Another area of application for machine learning is public safety. The iBorderCtrl project is currently developing a “smart” system to make border crossings at the Schengen external borders more effective. Machine learning is also used in this system, which consists of software and hardware components – such as scanners for the validation of ID documents or for the biometric identification of persons -: First, the relevant information is collected and processed digitally. This enables the systematic evaluation of border crossings and allows conclusions to be drawn, for example, about particularly high-risk groups of persons or newly emerging patterns when attempting to cross the border illegally. In this way, an individual risk value can be calculated in advance for each traveller and correspondingly more targeted controls can be carried out. The system learns from this, continuously improves itself and delivers ever more accurate results.
Discrimination through algorithms
From a legal point of view, such an approach entails various risks: First of all, the results always depend on sufficient data quality. Furthermore, algorithms can draw false conclusions and evaluate a risk greater than it actually is. Discrimination through algorithms cannot therefore be ruled out. Even if there were to be an overall increase in the efficiency of border controls, this would be in the public interest – faster and safer border controls – and in the interest of the individual.
The use of algorithms in public security in particular makes it clear that rules must be set in advance in order to avoid discrimination and lack of transparency. This also applies to the private sector. Within the framework of Mobilise – Mobile Man, scientists are therefore researching the requirements of algorithms. To date, not even the developers of self-learning systems have been able to understand exactly how the system arrived at a decision. If damage occurs, e.g. because the behaviour of man and machine diverges, it must be possible to understand what is happening. The question of who is responsible for damage caused by self-learning algorithms is also unclear. For these and many other reasons, ML will not only employ numerous lawyers, but will also bring about far-reaching changes in other areas, such as the insurance industry.
Featured Projects
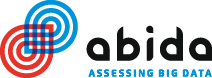
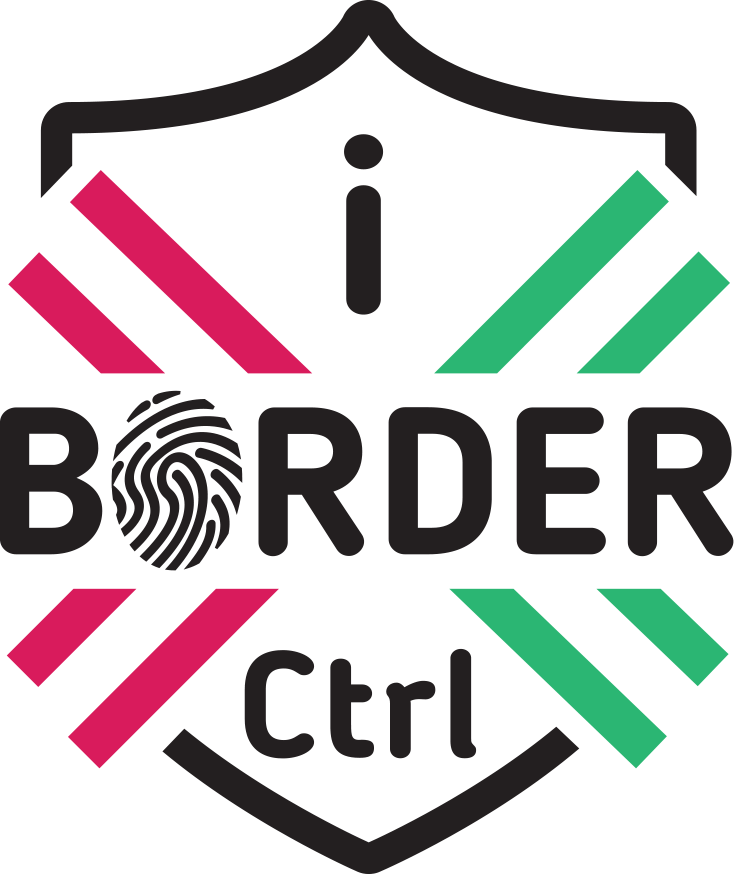
Contact
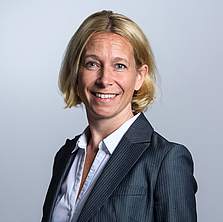
RAin Prof. Dr. Tina Krügel, LL.M
Tina Krügel is a lawyer and since April 2014 junior professor for information law, in particular data protection law, at Leibniz Universität Hannover. She has been a member of L3S since 2016.