Foto: ©whykei – unsplash.com
Autonomous driving under adverse conditions
Autonomous driving has made enormous progress in recent years. A number of companies are currently working on series maturity. Sensors must reliably detect the environment so that the self-controlling vehicle can travel safely. Laser, radar and camera systems are used. A digital model of the vehicle environment is created from the sensor data. Using this model, the vehicle is located on a highly accurate map, each static and dynamic obstacle is extracted and the route is planned based on this. Route planning also takes into account the predicted lanes of other road users in order to prevent collisions.
The system is not yet reliable: In the past, accidents have occurred when the environmental models created by the individual sensors contradict each other. In addition, driving in adverse weather conditions and (partial) sensor failures is currently only possible to a limited extent because not all information from the redundant sensors is yet combined.
What to do in bad weather?
In the BMWi-funded joint project ifuse, Prof. Dr. Holger Blume and his team are researching data structures, algorithms and architectures for an efficient fusion of raw sensor data. Compared to previous fusion techniques at object list level, the fusion of raw data enables more robust object classification and environment detection, even when individual sensors are affected. Sensor data fusion at raw data level is based on the signals of active and passive vehicle sensors, which are fused in a common coordinate system after minimal pre-processing. For each sensor value an additional confidence measure is calculated. Furthermore, the measurement inaccuracy of each measured value is explicitly modelled in the inverse sensor model and thus the greatest possible information content is used. The following AI-based algorithms recognize and classify those objects which are necessary for the planning of the journey. Based on the movement of an object, the future movement path is estimated and made available to path planning. At the end of the project, the research results will be presented in a test vehicle that will travel autonomously over a complex research intersection in Braunschweig.
Research vehicle generates training data
With the help of the own research vehicle PANDA (“PlAtform for the Development of Next-gen Driver Assistance”) data sequences are generated to train and validate the algorithms of machine learning. Future hardware architectures are emulated in the test vehicle in order to be able to guarantee secure and real-time processing of large amounts of data.
Contact
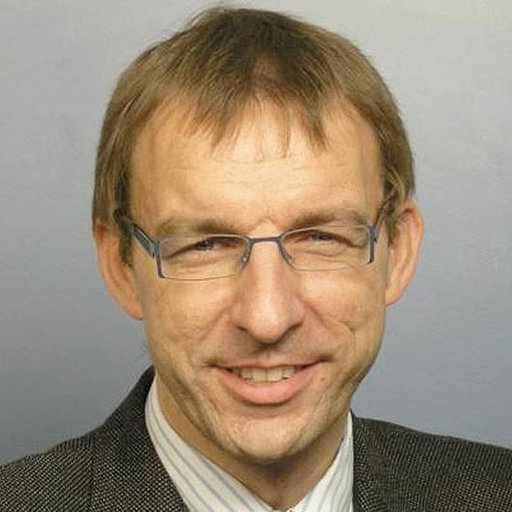
Prof. Dr. Holger Blume
L3S member Holger Blume heads the Department of Architecture and Systems at the Institute for Microelectronic Systems at Leibniz Universität Hannover and is project manager of ifuse.
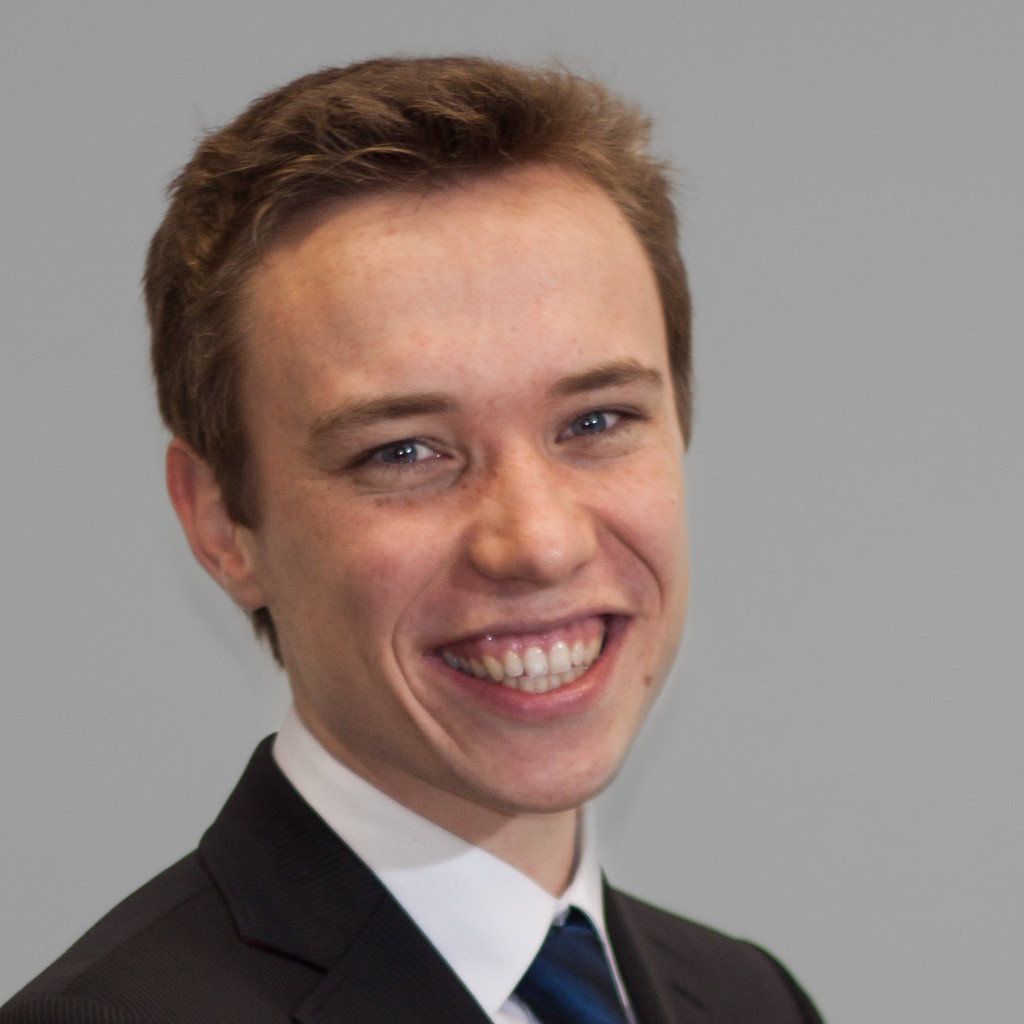
Nicolai Behmann, M.Sc.
Nicolai Behmann is a research assistant in the ifuse project. He deals with the fusion and interpretation of many sensor data with the help of artificial intelligence.