Foto: ©sp4764 – stock.adobe.com
Multifaceted collaboration: L3S and VW Commercial Vehicles
AI for Mobility and Production
From the offices of L3S on the fifteenth floor, the view extends to the Volkswagen plant in the north of Hanover. Physical proximity is not the only reason why VW Commercial Vehicles and the research center have been working together for a long time. The automaker and the L3S complement each other perfectly. “Silicon Valley has Stanford. We have the L3S with outstanding top researchers in AI and machine learning. We are taking advantage of this with the joint research projects. Why should the people at Stanford be smarter than those in Hanover?” says Dr.-Ing. Michael Nolting, Head of Digital Services & Data Analytics at Volkswagen Commercial Vehicles. The cooperation has resulted in the CampaNeo, d-E-mand and SmashHit projects, among others. VW mostly acts as a data collector, and L3S uses this data to create analyses and forecasts. Another issue in the projects is the traceability of data processing so that the respective project complies with the General Data Protection Regulation (GDPR).
Campaigns for data collection
In the CampaNeo project, the partners are working to build a platform that will enable private and public institutions to collect and analyze campaign-based vehicle data in real time. Based on the campaign data, VW and L3S also want to implement intelligent use cases.
For example, a campaign may aim to detect potholes. Drivers can join such campaigns and share their car data. For VW, the task is then to create an interface for communicating with drivers, collect the data and send it via a broker to the L3S data analysis infrastructure. There, scientists use the data for applications and analysis, improving data quality and opening up new applications, such as accident prediction. Kelvin Sopnan from VW is working with L3S scientist Rajjat Dadwal to make accident prediction even more accurate – with the help of various data attributes such as acceleration and braking signals, but also traffic lights, the road network and traffic-relevant locations such as schools or bus stops.
How should charging stations be distributed?
The prerequisite for the electric mobility turnaround is the development of a nationwide infrastructure and data-based services for all types of electric vehicles. In the d-E-mand project, solutions are being developed that can systematically predict and meet the demand for mobile and stationary charging infrastructure – especially during peak loads due to major events and mass movements such as the start of the vacations.
VW’s tasks include collecting the necessary data using vehicles equipped with sensors such as cameras or lidars. Using the data gathered, VW, with the support of L3S, is researching solutions that can forecast the need for charging stations.
Leonie Frantzen and Nicolas Tempelmeier from VW, together with Ashutosh Sao from L3S, are researching how charging stations can be optimally located in metropolitan areas. In doing so, they maximize the supply of charging infrastructure and minimize waiting, driving, and charging times while staying within budget constraints. The scientists achieve this with a novel deep reinforcement learning approach.
The trail of data
In the SmashHit project, the partners are working to make the exchange of data more secure and trustworthy. Both personal and industrial data are traded – the latter also across industries. The project partners want to develop a system that complies with the legal requirements of the DSGVO and uses newly developed security and data protection mechanisms to ensure data confidentiality and user trust.
In SmashHit, VW also acts as a data provider that wants to send data to an insurance partner, for example. This is to be done in a DSGVO-compliant manner and be transparent and traceable for the users, for example, car drivers. For this purpose, L3S is developing an infrastructure that enables seamless tracing of data (end-to-end traceability). Data suppliers and processors can use data traceability to verify compliance with legal requirements. Data owners can address their privacy concerns by being able to retrieve and trace their data via a central platform.
Nils Henke from VW and Stefan Schestakov from L3S are also researching how data can be generated that does not violate data protection laws. The idea is to use a data set to create a second, similar version that guarantees the data protection of the original and can be made available to third parties without hesitation. The approach is intended to facilitate access to such data, which has previously been difficult to share for data protection reasons.
AI in vehicle production
VW and the L3S are not only collaborating on mobility issues. They are also conducting joint research in the field of production. In the IIP-Ecosphere project, the partners are developing a demonstrator that will use AI to optimize cycle times across plants. The background: In industrial large-scale production, disruptions are associated with high costs. So far, research has focused on avoiding downtimes caused by standstills, for example through predictive maintenance. What is neglected are the very small delays in individual process steps. Such micro-disturbances are amplified throughout the entire process chain. As a result, delays that are initially minimal and hardly noticeable by the employee can end up causing high additional costs.
An interdisciplinary team from Volkswagen Commercial Vehicles, the L3S and the Institute of Manufacturing Engineering and Machine Tools at Leibniz Universität Hannover is developing an innovative, AI-based solution to this challenge: an intelligent manufacturing analysis system that monitors processes and optimizes cycle times by revealing cause-and-effect relationships of micro-disruptions. Volkswagen Commercial Vehicles is providing raw data and domain knowledge and is currently working to expand data collection. At L3S, Jeff Reimer is developing the methodology for automatic data interpretation and pattern recognition. He is also evaluating different deep learning architectures.
It is already possible today to collect and evaluate operating data in such a way that even the smallest deviations and their consequences in the manufacturing process can be identified. However, this requires time-consuming manual creation and maintenance of rules and regulations. The use of an innovative self-learning process data labeling with the help of clustering methods, combined with intelligent pattern and anomaly recognition in time series, should make this effort superfluous in the future. Advantage of the methodology: Adaptation to changed processes and transferability to other plants.
The method modules will initially be tested at Volkswagen Commercial Vehicles in Hanover. Later, the overall method is to be transferred to other plants. If this works, the final step will be to generalize the methodology so that the demonstrator solution can also be used for other applications. By integrating it into IIP-Ecosphere’s AI ecosystem, such as the IIP Platform and the AI Accelerator, other users will also have low-threshold access to this AI solution.
Contact
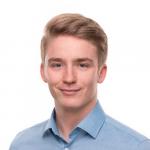
Stefan Schestakov
Stefan Schestakov is a PhD student at L3S and project leader for SmashHit. He conducts research in mobility, trajectories, and spatio-temporal prediction.
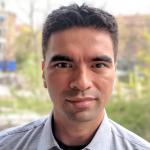
Jeff Reimer
Jeff Reimer is a PhD student at L3S and works within IIP-Ecosphere on the VWN demonstrator. His research addresses production, anomaly detection, and time series data.